The Overview
Submissions to this Challenge must be received on
February 28th, 2025
February 28th, 2025
Awards
The
total award pool
for this Challenge is
$50,000(USD)
$50,000(USD)
1st
Place
$30,000
Best
Execution
$15,000
Best
idea
$5,000
The Technology Innovation Institute (TII) launched the The
Crowd Label Challenge: Crowdsourcing LLM Feedback to find innovative ways for
humans to label data samples in a passive way.
Large language models (LLMs) utilize Reinforcement Learning
from Human Feedback (RLHF) to align intelligent agents with human preferences;
however, employing human labelers can be expensive.
TII has created an alignment samples database for use by LLM experts and
researchers to give scalable and reliable feedback to their models. It is now
interested in innovative passive human labeling methods where human users can
perform data sample annotation without even knowing they are completing
it.
TII is searching for approaches to present these alignment datasets to broad and
diverse audiences to label hundreds of thousands of data samples at scale and at
a lower cost.
Who Can Join?
The challenge is open to:
- Innovators
- Start-ups
- Research institutes
- University students from around the world
Eligibility
The Crowd Label Challenge: Crowdsourcing LLM Feedback invites innovators,
start-ups, research institutes, and university students from anywhere in the
world with the skills, resources and knowledge, who are all eligible to
participate in the Challenge, except:
- Employees of the TII and its affiliates; its parent company or other subsidiaries of the parent company;
- Employees of agents or suppliers of the TII or any of its affiliates, who are professionally connected with the Challenge or its administration;
- Members of the immediate families or households of the aforementioned;
- Any person or entity registered or ordinarily resident in a country that is on a sanctions list at any time during this Challenge (including, but not limited to, the Sanctions Lists maintained by the United States, the United Nations and the European Union).
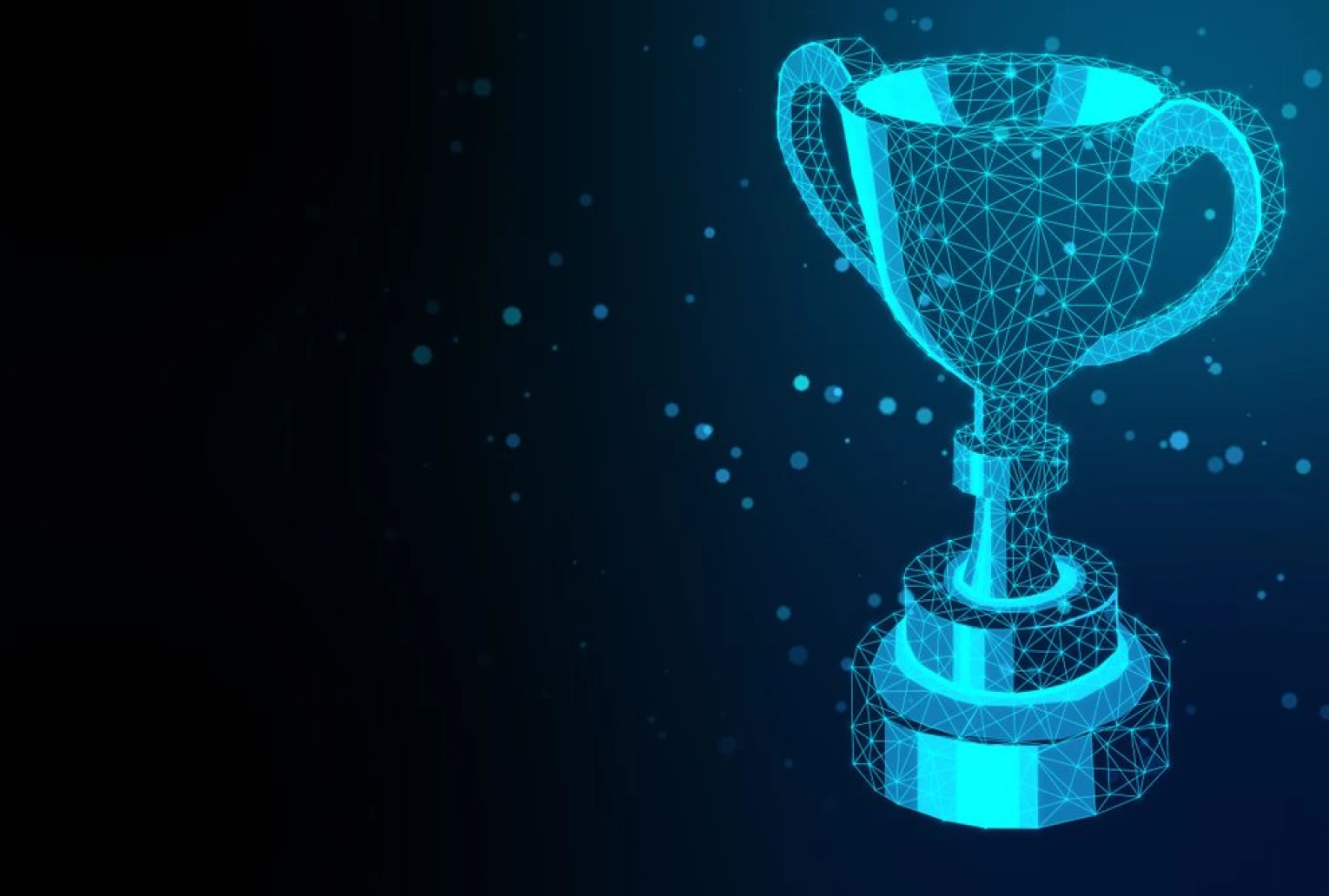
Timeline
*Evaluation period includes scoring/ranking, soliciting write-ups and Q&A from top
solvers,and final winner approvals for announcement.
Call for proposals
20/01/2025
Submission Deadline
14/03/2025
Finalists announcement and
development stage kick off
27/03/2025
Data collection stage kick off
(start of recording metrics for evaluation)
01/06/2025
End of data collection
30/06/2025
Winner annoucement (best overall
and best implementation)
31/07/2025
The Challenge
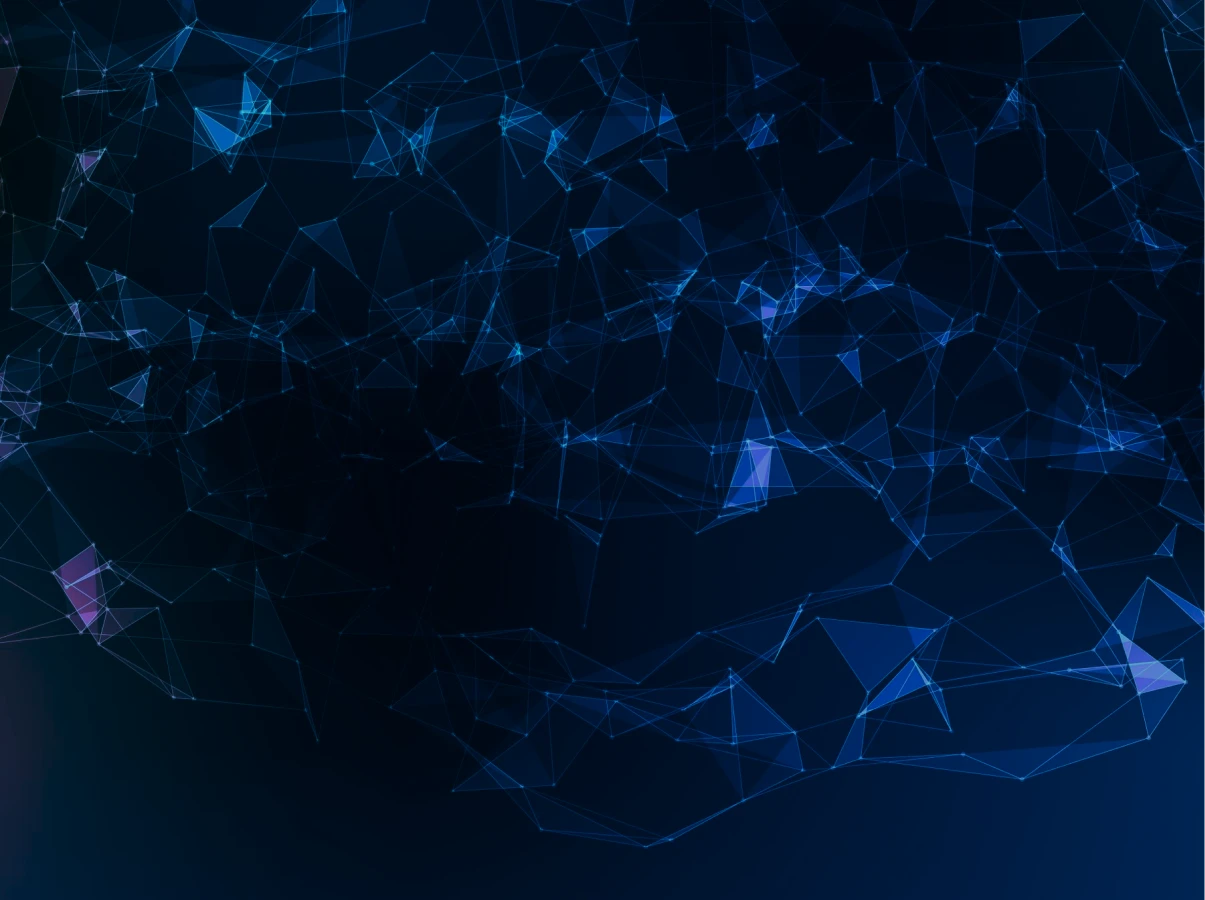
In this Challenge, TII is looking
for new and innovative ways to gather human labeling outputs in a passive
way, which would help LLM operators to scale their alignment efforts at much
lower costs.
The alignment must be achieved by
passive human labeling, where the task to label a data sample is so
ingrained into another process or operation that the human operator may not
realize they’re completing a labeling task.
In the development and deployment
of solutions and data collection stages, for shortlisted participants, it is
required that the passive human labeling method is tested and used by a wide
variety of users and experts. Giving details of the communities that are
being accessed to make the experimental launch a success is a key driver in
this Challenge.
The samples originate from a
controlled virtual domain deliberately designed to source and enhance
different types of samples that may be used in LLM alignment by TII. These
can include visual question answering (VQA) samples. By manipulating visual
complexity and conceptual distributions, VQA samples provide a diverse and
robust testbed for models aiming to excel in visual reasoning.
Key Features of
VQA Samples
Rich Visual
Details Each scene is composed of objects with intricate part-based attributes, arranged in various configurations that challenge the models to accurately perceive and interpret the visual content.
Details Each scene is composed of objects with intricate part-based attributes, arranged in various configurations that challenge the models to accurately perceive and interpret the visual content.
Comprehensive
Question Sets Each scene is composed of objects with intricate part-based attributes, arranged in various configurations that challenge the models to accurately perceive and interpret the visual content.
Question Sets Each scene is composed of objects with intricate part-based attributes, arranged in various configurations that challenge the models to accurately perceive and interpret the visual content.
Multilingual
Aspect The sample database includes multiple languages. This feature encourages cross-lingual adaptability of solutions−the method by which it can provide language samples to users who speak that language−and expands the criteria for evaluation.
Aspect The sample database includes multiple languages. This feature encourages cross-lingual adaptability of solutions−the method by which it can provide language samples to users who speak that language−and expands the criteria for evaluation.
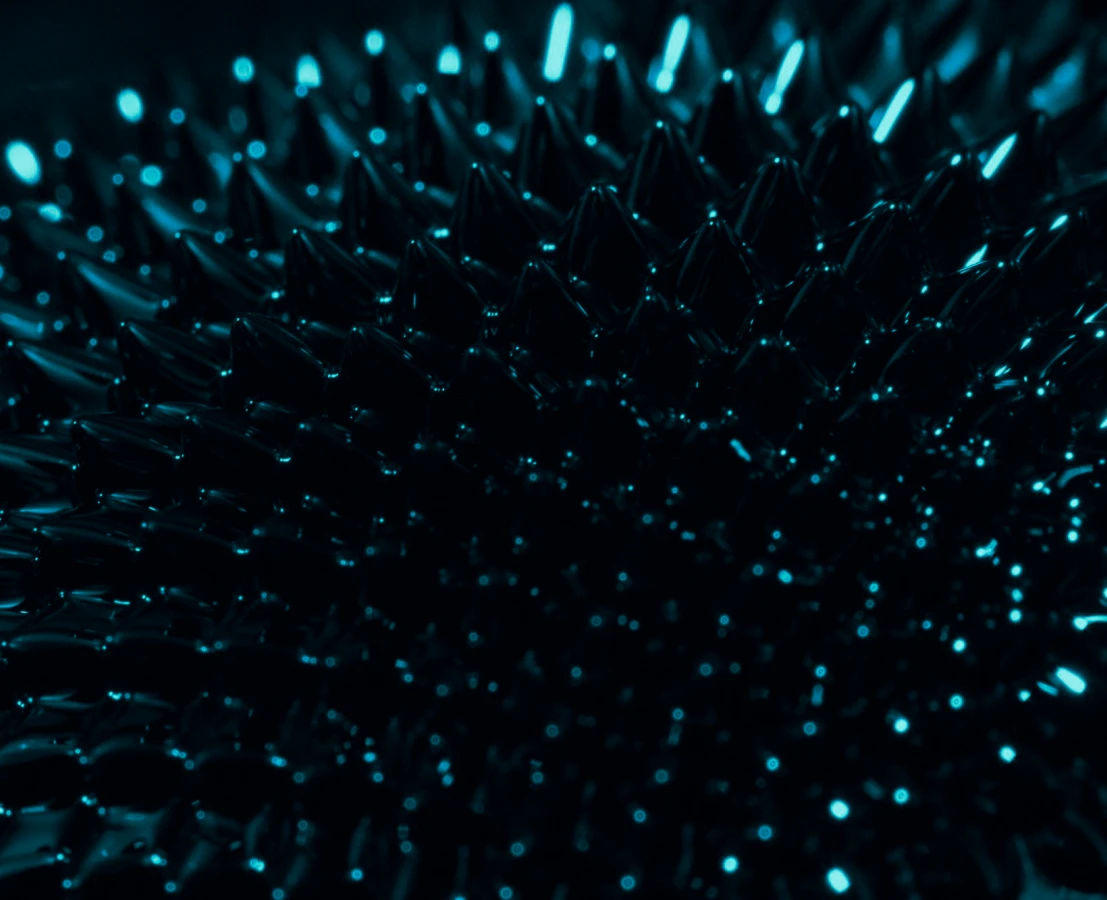
The passive human labeling methods
must distribute data samples tailored to individual users’ expertise or
preferences. Personalization based on topic, mode, language, or complexity
is a key requirement of the Challenge.
There is a lot of room for
innovation, as said methods need to incentivize people to use them
passively, as well as be able to target different groups with different
topics and different labeling complexity levels.
Submissions would be evaluated
based on creativity, scalability, the feasibility of the idea, business
model feasibility, potential accuracy of the resulting labeling, and
forecasted costs.
Check all the Challenges
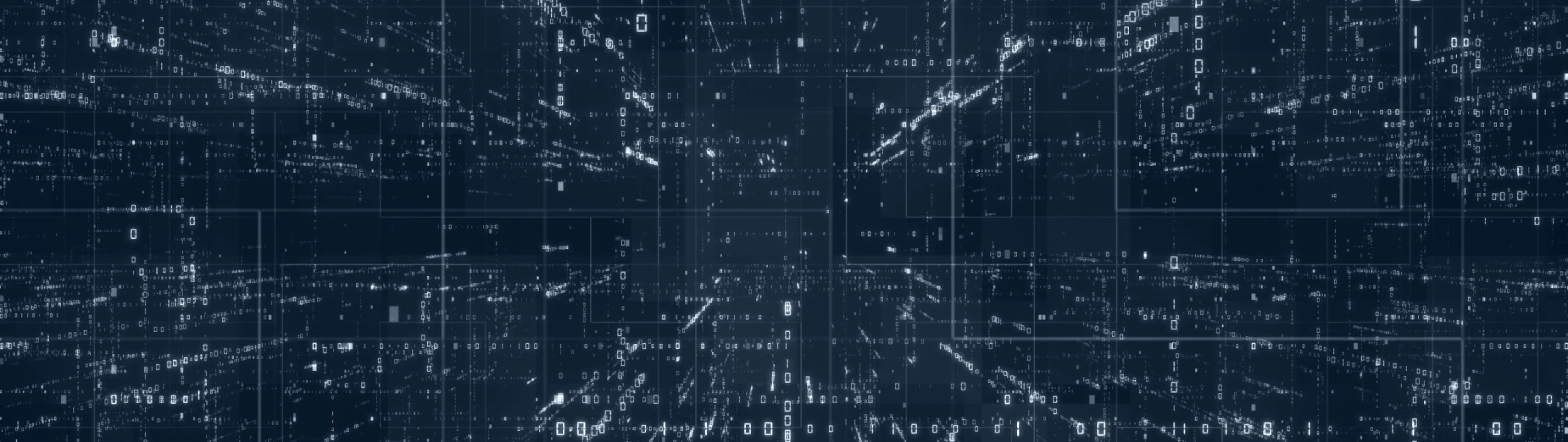